Shaping the Future with Generative AI
The data science behind Rayven AI, especially in the context of generative AI and real-time data, encompasses several advanced techniques and methodologies aimed at extracting insights, making predictions, or generating content from live data streams
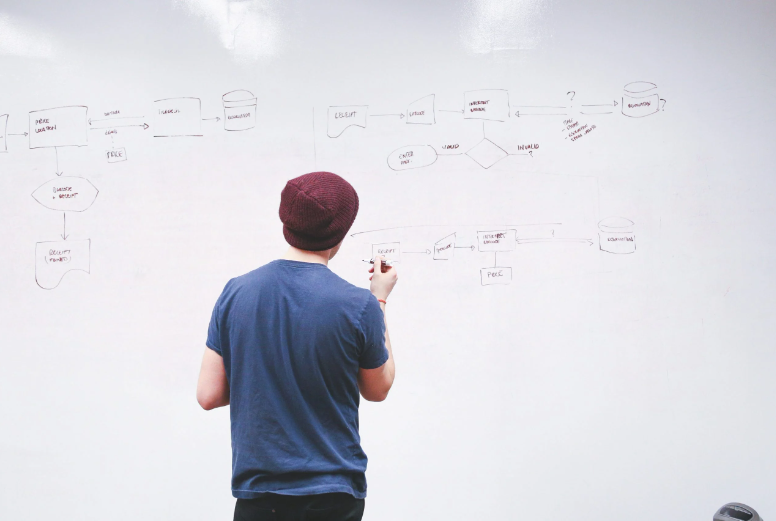
Everting you need in one fully integrated enterprise Genitive AI platform
Our platform has all the layers needed to deploy your Genrative AI solution.
Data Collection and Preparation Layer
Data Sourcing: Collecting a vast and diverse dataset from books, websites, articles, and other text sources.Data Cleaning: Removing or correcting inaccuracies, biases, and inappropriate content from the data.Tokenization: Breaking down the text into smaller units (tokens), such as words or subwords, for processing.
Model Architecture Layer
Embedding Layer: Converts tokens into vectors (numerical representations) to capture semantic meanings. Transformer Layers: The core of modern LLMs, consisting of self-attention mechanisms and feed-forward neural networks that process the input sequentially and in parallel, allowing the model to weigh the importance of different words relative to each other.
Training Layer
Objective Functions: Defines the goal the model aims to achieve, such as minimizing the difference between the predicted text and the actual text.Optimization Algorithms: Algorithms like Stochastic Gradient Descent (SGD) or Adam are used to adjust the model's parameters to minimize the objective function.
Regularization and Normalization Layer
ropout: Randomly drops units from the neural network during training to prevent overfitting.Layer Normalization: Standardizes the inputs to each layer for each mini-batch, helping to stabilize the learning process.
Fine-tuning and Evaluation Layer
Fine-tuning: Adjusting the model on a smaller, domain-specific dataset to improve its performance on specific tasks.Evaluation Metrics: Measures like perplexity, BLEU (Bilingual Evaluation Understudy) score, or ROUGE (Recall-Oriented Understudy for Gisting Evaluation) score are used to evaluate the model's performance in terms of fluency, coherence, and relevance of the generated text.
Deployment and Scaling Layer
Model Compression: Techniques like quantization and pruning are used to reduce the model's size for easier deployment.Serving Infrastructure: Systems and APIs that allow the model to be accessed by users, applications, or other systems in real-time or batch processing modes.
Pre-training Customization Layer
Curriculum Learning: Gradually increasing the difficulty of the training data or tasks, allowing the model to first learn simpler patterns before tackling more complex ones.Data Augmentation: Enriching the training dataset by modifying existing examples or generating new ones to improve the model's robustness and its ability to generalize from the training
Post-training Optimization Layer
After the model has been trained, further optimizations can be applied to ensure the model's scalability, efficiency, and deployment readiness. This layer includes:Quantization: Reducing the precision of the model's parameters (e.g., from 32-bit floating points to 16-bit or 8-bit), which can decrease the model's size and speed up inference while maintaining performance.
Real-Time Data Processing
Stream Processing: Real-time data, such as from IoT devices, social media feeds, or online transactions, requires technologies capable of processing large volumes of data quickly. Tools like Apache Kafka or Amazon Kinesis are often used to ingest, process, and analyze data streams on the fly.
Data Ingestion and Normalization: Real-time data must be cleaned and normalized as it's ingested. This involves removing duplicates, handling missing values, and converting data into a uniform format for analysis.

Machine Learning and Predictive Analytics
Real-Time Predictive Models: Data science in this context often involves building machine learning models that can predict outcomes based on incoming data. These models are trained on historical data but are applied in real-time to make predictions about new data.
Adaptive Learning: Models may need to adapt to changes in data patterns over time. Techniques like online learning or continual learning allow models to update themselves as new data comes in, ensuring predictions remain accurate. higher efficiency, reduce operational costs, and provide better services to their customers.
Rayvens' platform facilitates these advancements by offering a scalable, customizable, and easy-to-integrate solution that addresses the specific challenges and opportunities in these sectors.

Generative AI
Generative Models: Generative AI involves models that can generate new data points, simulations, or content that resembles the training data. In the context of Rayven AI, this could involve generating synthetic data for simulations, creating realistic scenarios for training, or developing new designs or patterns.
Applications in Real-Time: When combined with real-time data, generative AI can provide predictive simulations that adapt to changing conditions, generate content based on current trends, or create data-driven recommendations instantaneously.

Analytics and Visualization
Real-Time Dashboards: Data visualization tools and dashboards are essential for presenting the insights derived from real-time data and generative AI models. These tools often provide interactive and live-updating visualizations that help in monitoring performance, trends, and anomalies.
Decision Support: The insights gained from analyzing real-time data with generative AI models can inform immediate decision-making. For instance, in a manufacturing context, this might involve adjusting processes in response to live performance data or predictive maintenance alerts. also contribute to sustainability goals by reducing fuel consumption and promoting recycling.

Challenges and Solutions
Latency and Scalability: Handling real-time data with low latency and high scalability is a challenge. Solutions often involve distributed computing and specialized hardware like GPUs for intensive computations.
Data Privacy and Security: Real-time and generative AI applications must address data privacy and security, especially when dealing with sensitive information. Techniques like federated learning or differential privacy can help mitigate these concerns.

Configure, build the logic, features + intelligence that runs your custom applications.
Get our free ebook on how you can extract real-time metrics and predictive insights from all your data sources, and create Generative AI custom solutions, simply.
Get our free ebook on how you can create integration, real-time data + Generative AI solutions to improve your business
Why Developers and Data Professionals Choose Rayven
-
Low Code
-
Flexibility
-
Robust Features and Tools
-
Security and Compliance
Easy-to-use, drag-and-drop low code configuration
Easy-to-use, drag-and-drop configuration.
Rayven's user-friendly interface and simplified processes, significantly reduces development time and effort. Intuitive design and ability to start projects quickly without a steep learning curve.
Powerful Customization and Flexibility capability
offer high levels of customization and flexibility are often favored by developers. If Rayven.io allows users to tailor their environment, tools, or workflows to their specific needs, it could be a significant reason for its popularity.
Comprehensive set of features and tools all in one integrated platform
A comprehensive set of features and tools that cater to developers' needs—such as debugging tools, project management features, or integration capabilities with other software makes the platform stand out. Rayven.io offers unique or superior functionalities compared to other platforms from data integration, workflows AI and more...
Security and Compliance all built in
Given the increasing importance of cybersecurity and data privacy, Rayven offers robust security features and help developers meet compliance requirements.
Common pain-points solved using our technology include:
Data is siloed +can’t be analysed
Rayven, a company specializing in IoT (Internet of Things) and AI (Artificial Intelligence) solutions, addresses the common issue of data being siloed and the inability to analyze it in real-time through a few key approaches:
Unified Data Ecosystem
Rayven creates a unified data ecosystem by integrating various data sources, including IoT devices, cloud services, and existing databases, into a single platform. This integration breaks down the silos that typically isolate data, making it difficult to access and analyze collectively.
Real-time Data Processing
By leveraging IoT technology, Rayven can collect data in real-time from a wide array of devices and sensors deployed across different locations and operations. This capability is crucial for industries that rely on timely data to make informed decisions, such as manufacturing, agriculture, and energy.
Advanced Analytics and AI
Rayven employs advanced analytics and AI algorithms to analyze data as it is collected. This real-time analysis enables immediate insights, which can be used to optimize operations, predict maintenance needs, and improve overall efficiency. The AI component learns from data patterns over time, enhancing its predictive capabilities and providing more accurate insights.
User-friendly Interface
To make real-time data analysis accessible to all levels of users, Rayven provides a user-friendly interface that simplifies the visualization and interpretation of data. Users can customize dashboards to display relevant metrics and KPIs (Key Performance Indicators), enabling them to monitor performance and make data-driven decisions without needing deep technical knowledge.
Scalability and Flexibility
Rayven's platform is designed to be scalable and flexible, accommodating the growth of an organization's data needs. This design ensures that as more devices are connected and more data is generated, the system can scale without compromising performance or the ability to analyze data in real-time.
Legacy systems are difficult to integrate
Integrating legacy systems into modern data and AI platforms like Rayven.io can be challenging due to a variety of factors, including outdated technology, lack of support for modern integration protocols, and complex, bespoke configurations. However, platforms designed to facilitate the use of generative AI and other advanced data analytics techniques typically employ several strategies to overcome these challenges and successfully integrate with legacy systems. Here's how Rayven.io or similar platforms might approach this issue:
1. Customizable Connectors
Description: Customizable connectors are designed to interact with the specific protocols and data formats used by legacy systems. Even when a system uses outdated or proprietary communication protocols, these connectors can be tailored to facilitate data extraction and ingestion.
Benefit: They enable seamless data flow from legacy systems to the platform without requiring significant changes to the existing infrastructure.
2. API Wrappers
Description: API wrappers act as intermediaries that translate between modern APIs and the interfaces provided by legacy systems. They can encapsulate the complexity of direct communication with legacy systems and offer a more modern, RESTful API interface to interact with.
Benefit: This method allows developers to work with familiar technologies and integration patterns, reducing the learning curve and development effort.
3. Middleware Solutions
Description: Middleware acts as a bridge between legacy systems and new platforms, offering a range of services such as message queuing, data transformation, and application integration. It can handle the intricacies of communication between systems of different ages and capabilities.
Benefit: Middleware can greatly simplify the integration process, providing a robust and flexible way to connect disparate systems.
4. Data Transformation and Normalization
Description: Once data is extracted from legacy systems, it often needs to be transformed or normalized to match the format and structure expected by modern data analytics and AI models.
Benefit: This step ensures that data from older systems can be effectively used alongside data from newer sources, maintaining the integrity and consistency necessary for accurate analysis and model training.
Reporting is manual, out of date, or best guess
1. Automation of Data Collection and Integration
How it Works: Automating the collection and integration of data from various sources eliminates the need for manual data entry, which is time-consuming and prone to errors. By using connectors or APIs to automatically fetch data from systems, devices, and other data stores, Rayven.io ensures that the data feeding into the reporting system is current and comprehensive.
2. Real-time Data Processing
How it Works: Implementing real-time data processing capabilities allows the system to update reports as soon as new data arrives. This means that reports reflect the most current state of affairs, rather than being a snapshot of the past. For businesses, having access to real-time information can be critical for making timely decisions.
3. Advanced Analytics and AI
How it Works: By applying advanced analytics and AI models, Rayven.io can analyze historical data to identify trends, patterns, and correlations. This capability allows for the generation of predictive insights, moving beyond what has happened to what is likely to happen in the future. Reports can include forecasts and recommendations, making them more valuable for decision-making.
4. Customizable Dashboards and Reporting Tools
How it Works: Offering customizable dashboards and reporting tools enables users to create reports that align with their specific needs and preferences. Users can select the metrics that matter most to them, design reports that highlight critical information, and automate the generation and distribution of these reports. This flexibility ensures that reports are relevant, useful, and tailored to the audience.
5. Collaboration and Sharing Features
How it Works: Facilitating easy sharing and collaboration on reports ensures that all stakeholders have access to the latest information. By supporting different formats and channels for report distribution (e.g., web dashboards, PDFs, email alerts), Rayven.io ensures that insights reach the right people at the right time, enabling faster and more informed decision-making.
Machine Learning +AI adoption is a pipe dream
1. Complexity of Data Integration
Challenge: Organizations often struggle with integrating disparate data sources and ensuring data quality.
Solution: Rayven.io provides a unified platform for integrating data from any asset, system, device, or data store. This helps create a single source of truth, simplifying data management and making it easier to prepare data for AI and ML models.
2. Technical Expertise Required
Challenge: Developing and deploying AI and ML models typically requires specialized knowledge, which can be a barrier for many organizations.
Solution: Rayven.io likely offers user-friendly interfaces, pre-built models, and automated workflows that abstract the complexity of AI and ML development. This democratizes access to AI technologies, allowing users with varying levels of expertise to create and use AI solutions.
3. Model Training and Deployment
Challenge: Training AI and ML models can be resource-intensive and time-consuming, and deploying them into production environments can be complex.
Solution: The platform may offer scalable cloud-based infrastructure that automates the model training and deployment processes. This can significantly reduce the time and resources required, making it more feasible for organizations to adopt AI and ML solutions.
4. Security and Compliance
Challenge: Ensuring data privacy, security, and compliance with regulations can be daunting when implementing AI and ML solutions.
Solution: Rayven.io is designed with security and compliance in mind, providing features that help organizations manage their data securely and adhere to regulatory requirements. This alleviates concerns about data breaches and privacy violations.
5. Scalability and Performance
Challenge: As organizations grow, their AI and ML solutions need to scale accordingly, which can be challenging to manage.
Solution: The platform offers scalable solutions that grow with an organization's needs, ensuring that AI and ML applications perform optimally even as data volumes and user demands increase.